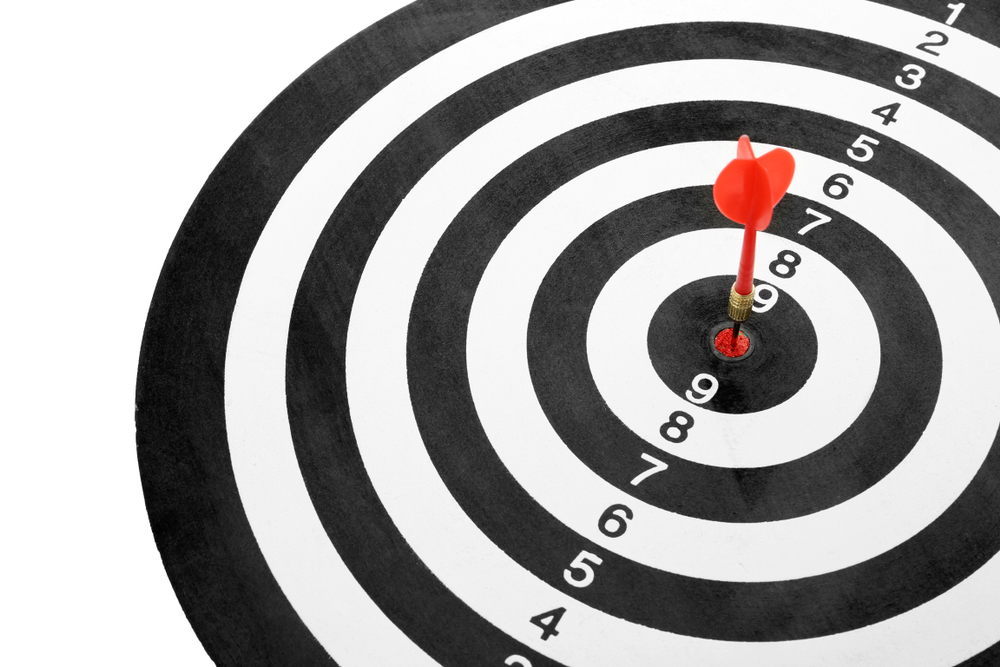
At one of the last conferences I attended, one of the attendees earnestly asked the speaker, “what do we need to do to have successful Marketing AI projects?”
The speaker, who apparently thought he was auditioning for an episode of Snark Tank, retorted, “learn how to measure them properly,” without even a chuckle.
The attendee didn’t move from the microphone at the front, waiting patiently for a “real” answer.
The speaker, whom I consider to be one of the biggest all-hat-no-cattle guys on the circuit, looked at him point blank and said: “that’s my final answer.”
Looking dejected, the attendee moved on.
You’ll see a version of this at many AI conferences, webinars, and Zoom meetings these days. And while the All Wax No Wick speaker was his usual caustic burro self, he was right that if you want to grow your revenues using AI, it’s critical to evaluate all your AI/ML marketing projects. More importantly, you need to figure out how you are defining success.
Just like in the early days of “WORLD WIDE WEB” projects, you’re going to want to look at three main things: (1) if the technology itself is working; (2) whether you’re hitting the desired outcome that you set before you began the project; and (3) what the potential is for the future.
You already know that AI/ML is not a set-it-and-forget-it thing. What you may not know is that sometimes a project will work like gangbusters, but during the process, you’ll realize that you have a better way to approach the problem or that perhaps it’s just not prudent to run it long-term. (Think projects with huge bias swings/tendencies.) AI learns and (hopefully) gets smarter as it does, so it’s important that we hold space for growing along with it.
Let’s go through these each of the questions one by one.
IS THE TECHNOLOGY WORKING?
These days, AI vendors commonly get their systems into clients with the “your first x projects are free” approach. This allows clients to test-drive the software/technology/algos for real, instead of just watching a demo or reading a kinda-sorta-but-not-really-the-same case study. Frankly, the whole “free x” typically works out great, and… Whether you’re using in-house or outside technology, after every project, you should ask yourself is the technology working? What do we need from the algo/software/package/system/etc. that we don’t currently have? What existing benefits are we not using that we should be? How do we get more bang for our buck out of our current setup? Are we seeing anything concerning with the technology? (Privacy concerns? Security concerns? Inherent bias?)
ARE WE HITTING THE DESIRED GOAL/OUTCOME?
After you roll through the questions about the systems part of the technology, you’ll want to ask yourself whether you met your business objective(s) by doing the project. Every company sets its desired outcomes a little differently. Some measure success by revenue. Others measure success by how much a specific metric changed positively or negatively. (For example, decreasing complaints.) Many eCommerce folks look at improving overall conversion. (Hint: focusing on adoption is often more productive.) Many companies look at productivity metrics or how much time/money/resources they saved.
Whatever goals you identified at the beginning of your project is what you should measure. If you want to add additional items, that’s cool too but be sure to document the project as it was intended. Having a historical record of all your AI successes and failures is especially useful in AI, where the technology is still growing by leaps and bounds. And yes, I know how tempting it is to change the goals mid-project, especially when you’ve had one too many failures in a row but stay strong and don’t do it. Report on your original intent and then add the good stuff as a bonus. Things get easier as you get more projects under your belt, I pinky-swear promise.
WHAT IS THE POTENTIAL FOR THE FUTURE?
Many folks skip this part, but I find it most fruitful. There are two parts to this: (1) Is whatever you set out to do being used the way you expected and/or resulting in the correct action(s) and (2) how to do we best roll it out (or not?)
Take Personalized Product Recommendations, for example. Say I wanted to implement dynamic recommendations in my dropcart with a 10% click rate. (Conservative, I know.) After the project was over, I found that I got a 17% click rate. Did I set out to do what I wanted? Yes! Score 1 for me. Is the use of AI resulting in the right action? Yes, for increasing click rate! What would you say if I told you I got the 17% clickthrough but nary an order? What would you think then? Did the project work? Perhaps. If my goal is/was to increase page depth or AAUS (Active Average User Session), it worked. If my long-term goal is to increase revenues? Well, then, I still have more work to do.
You might say you’d never set short-term goals without looking at the long-term position. What happens if you get the 17% click rate and a 6% adoption to cart rate overall, but the products that work best in the dropcart all sell out? What happens if adding the additional item pushes a percentage of the users into the next shipping bracket, and you end up with lower overall conversion and, more importantly, less revenue than you did before? Still a win?
You can say that you’ll accommodate for every possible scenario, and I’m sure it’s possible but not all that probable, especially since the example I used is oversimplified. One of the most significant benefits of AI is that it can find things you don’t. Plus, those scenarios become more complex and often surprising as it gets more sophisticated. (Surprising in a good way, not a scary clown way.)
After you figure out whether what you did worked (or not), you’ll want to develop a plan for future rollout (or not.) What’s the next step? Do you need to make any tweaks to the system? Are you uncovering any biases that need to be addressed? Is all your data still in good shape? In retrospect, did you train your model(s) correctly? Is there anything you need to disrupt? Do you need to loop in anyone else on the results you’re seeing — Operations, Purchasing, or Customer Service, perhaps? Any costs/expenses/funding/additional support you need to discuss? What can you do to increase your ROI? Any new measures you need to communicate? (This one is often overlooked, but it’s essential.) Implementing AI/ML gives us new things to look at – and new ways to look at them. If you’re finding those types of things, identify them and then measure, report, and communicate them properly.
Artificial Intelligence has been around since the 1950s, and its growth, especially in Marketing, has skyrocketed in the last decade. There’s no magic formula for having the perfect project. With that said, you can stack the deck for success by ensuring the project will benefit from AI; getting your data in tip-top shape; developing a solid team (including your outside vendors); setting clear expectations, and measuring and communicating your success to the organization.
What else? Anything you’d like to add? Have a tip you’d like to share? Tweet @amyafrica or email me at info@eightbyeight.com
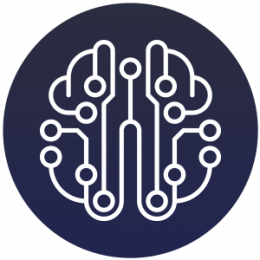